An Introduction to Statistical Learning: with Applications in R (Springer Texts in Statistics)

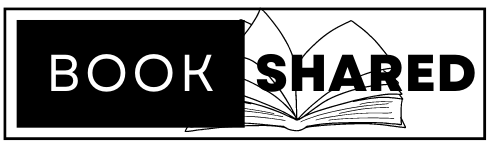
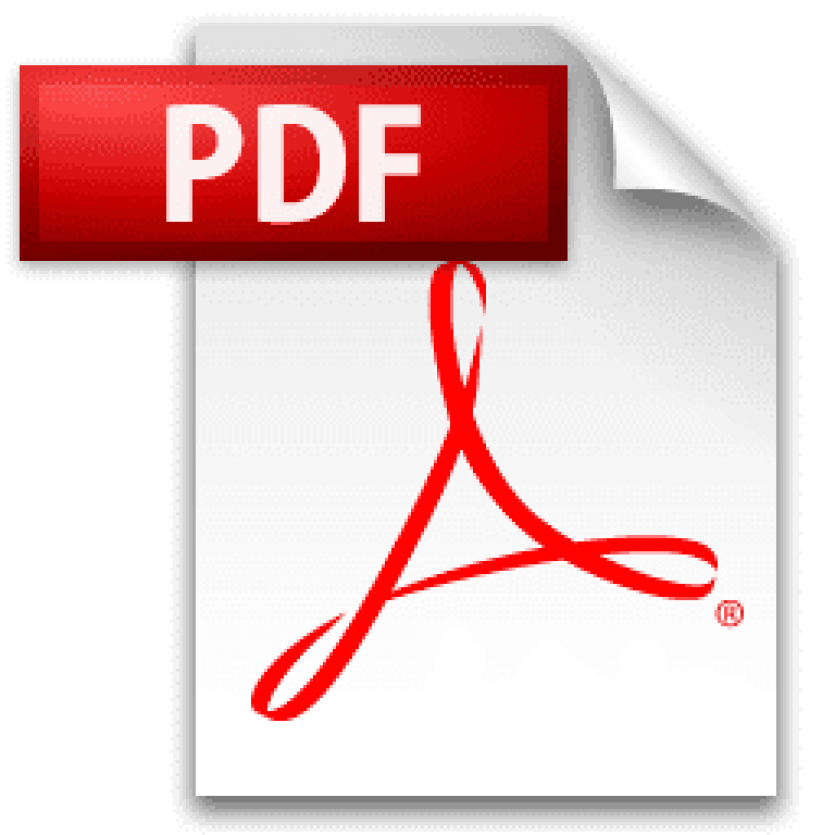
Note: If you encounter any issues while opening the Download PDF button, please utilize the online read button to access the complete book page.
Size | 26 MB (26,085 KB) |
---|---|
Format | |
Downloaded | 654 times |
Status | Available |
Last checked | 13 Hour ago! |
Author | Gareth James |
“Book Descriptions: An Introduction to Statistical Learning provides an accessible overview of the field of statistical learning, an essential toolset for making sense of the vast and complex data sets that have emerged in fields ranging from biology to finance to marketing to astrophysics in the past twenty years. This book presents some of the most important modeling and prediction techniques, along with relevant applications. Topics include linear regression, classification, resampling methods, shrinkage approaches, tree- based methods, support vector machines, clustering, and more. Color graphics and real-world examples are used to illustrate the methods presented. Since the goal of this textbook is to facilitate the use of these statistical learning techniques by practitioners in science, industry, and other fields, each chapter contains a tutorial on implementing the analyses and methods presented in R, an extremely popular open source statistical software platform. Two of the authors co-wrote The Elements of Statistical Learning (Hastie, Tibshirani and Friedman, 2nd edition 2009), a popular reference book for statistics and machine learning researchers. An Introduction to Statistical Learning covers many of the same topics, but at a level accessible to a much broader audience. This book is targeted at statisticians and non-statisticians alike who wish to use cutting-edge statistical learning techniques to analyze their data. The text assumes only a previous course in linear regression and no knowledge of matrix algebra.”